Synthetic ML Data for Urban Planning, Architecture Visuals
City Digital Twins Help Train Deep Learning Models to Separate Building Facades
Tags: Osaka University, Japan, Construction & Smart Cities
Osaka University researchers have innovated a method combining digital twins of cities, created using 3D models and game engines, with real city images to generate training data for deep learning models, enhancing architectural analysis. This approach facilitates the automatic separation of building facades in images, aiding construction management, architectural design, and energy analysis. Utilizing the Unity game engine and real street-level images, they created a hybrid dataset that improves the accuracy of deep convolutional neural networks (DCNNs) in architectural segmentation. Their findings show that synthetic data can potentially replace real images in training sets, offering a cost-effective solution for training DCNNs. This technology finds applications in construction management, retrofit measurements, and architectural visualization.
IP Type or Form Factor: Software & Algorithm
TRL: 4 - minimum viable product built in lab
Industry or Tech Area: Smart Cities & Urban Planning; Architecture & Interior Design
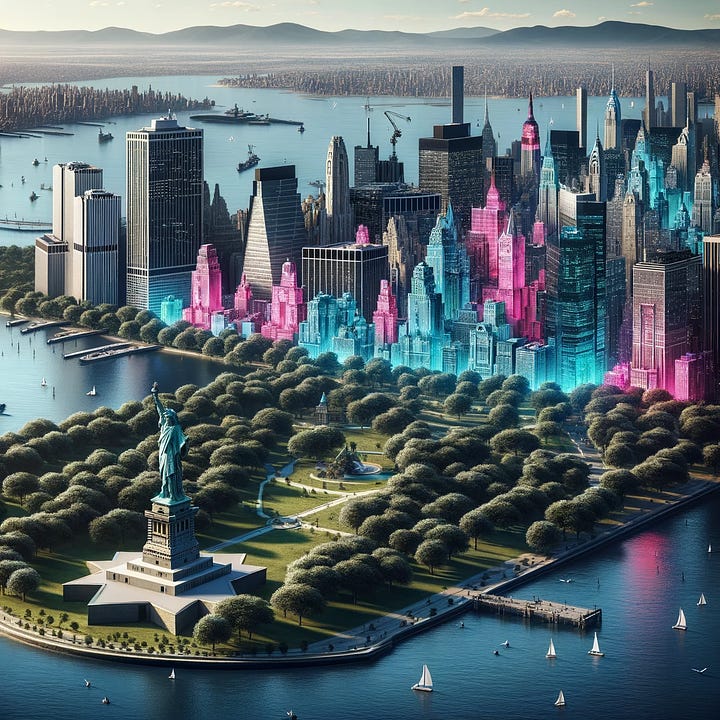
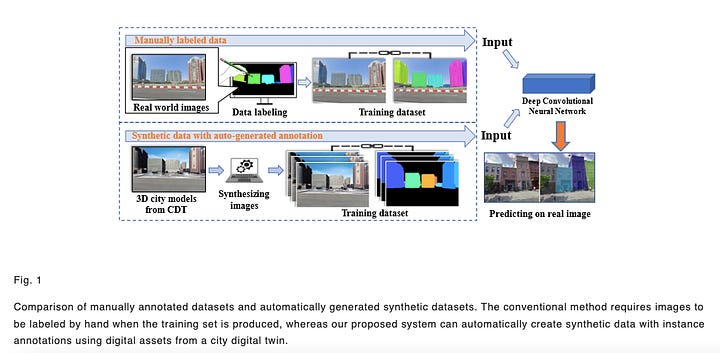
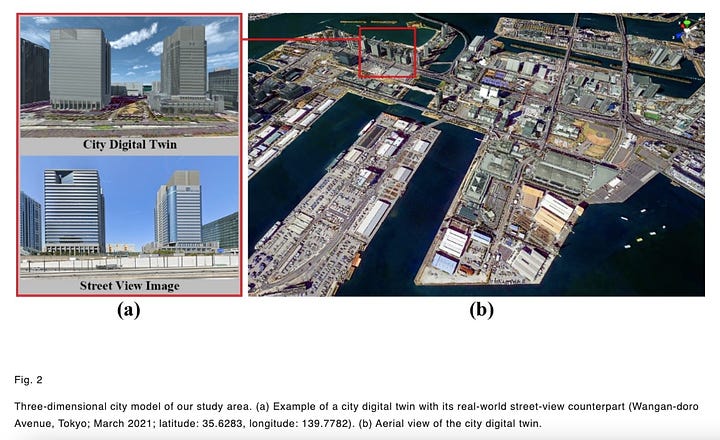
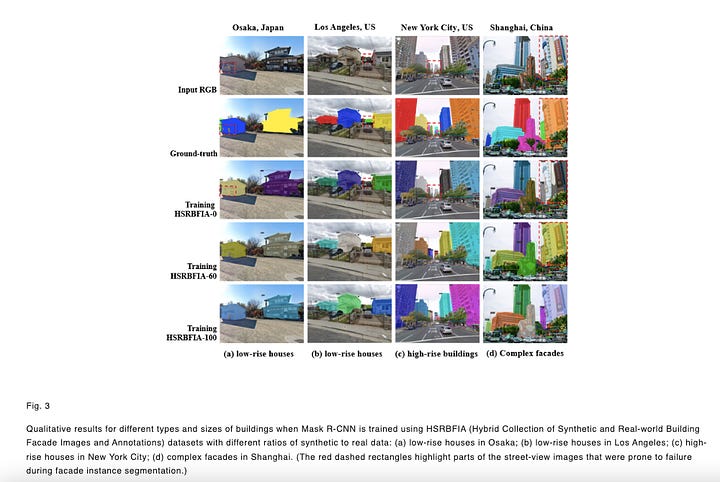