Optimized Routes and Job Allocation in Urban Delivery
AI-Based Dynamic Route Optimization and Driver Job Recommendation Tool
Tags: Singapore Management University, Singapore, Infrastructure, Utilities & Communications, Industrial Automation & Manufacturing
This AI-based tool uses dynamic route optimization and job recommendation algorithms to improve efficiency in same-day eCommerce logistics, addressing the challenges of urban delivery operations. Built on SMU's Collaborative Urban Delivery Optimization (CUDO) technology, it assigns delivery orders to drivers based on travel distance and order status, maximizing rider earnings and minimizing delivery time. The system’s reinforcement learning algorithm adapts to optimize job recommendations and dispatches, benefiting logistics providers, drivers, and customers alike. Applications include same-day delivery optimization, logistics planning, and reducing carbon footprints through efficient routing. A pilot test showed a 20% increase in efficiency, saving 500 delivery hours.
IP Type or Form Factor: Software & Algorithm
TRL: 6 - prototype validated in intended environment
Industry or Tech Area: Logistics; Internet of Things
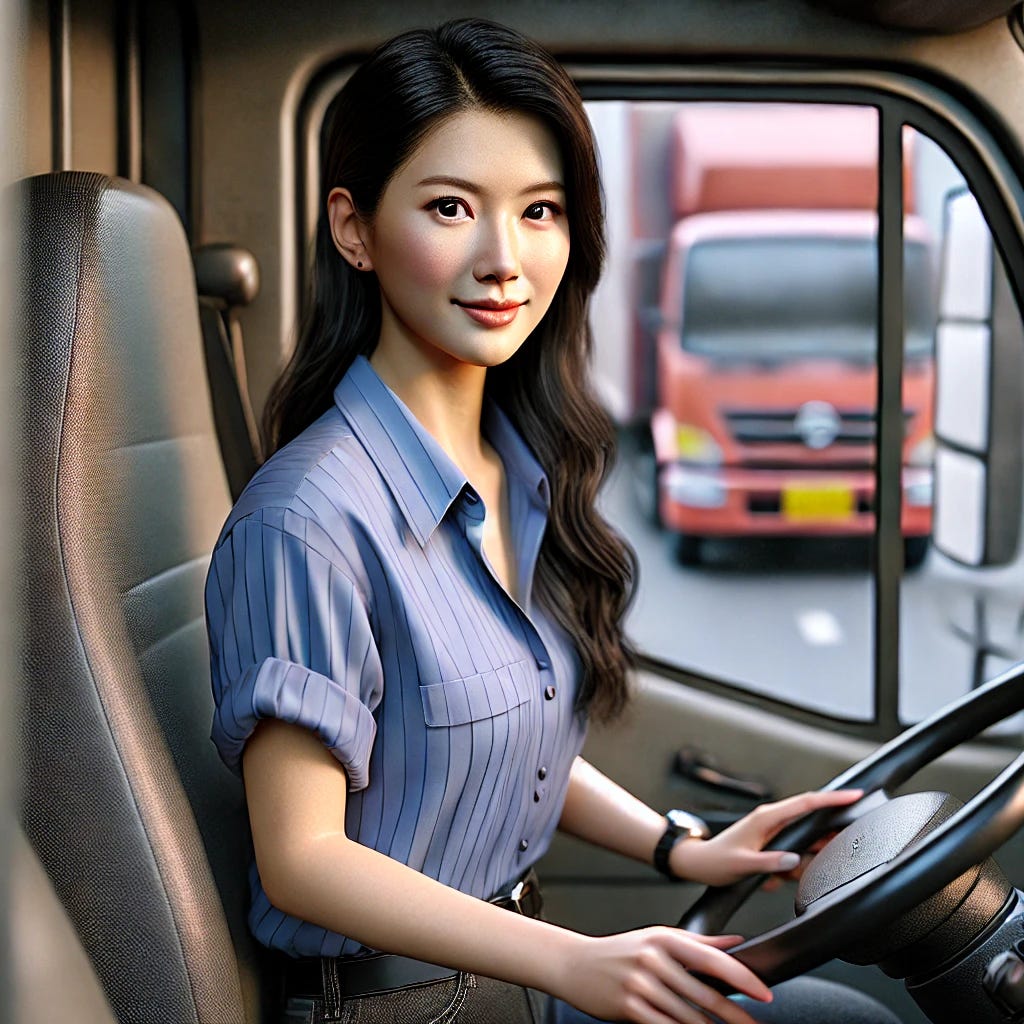