ML Wind Speed Prediction for Optimizing Renewable Energy
Wind Speed Prediction Over Malaysia Using Various Machine Learning Models: Potential Renewable Energy Source
Tags: Universiti Malaya, Malaysia, Energy & Environment, Computing Technology
This study applies machine learning models, including Gaussian process regression (GPR), bagged regression trees (BTs), and support vector regression (SVR), to predict wind speed in Malaysia. Using 19 years of data from 14 meteorological stations, the GPR model was found to provide the most accurate predictions for weekly wind speeds. The results aid in optimizing wind energy systems by forecasting wind behavior, a critical factor for renewable energy development. The GPR model outperformed SVR and BTs in most cases, except for a few stations. This research supports the potential of machine learning in enhancing renewable energy planning in Malaysia.
IP Type or Form Factor: Discovery & Research; Software & Algorithm
TRL: 5 - prototype ready for testing in intended environment
Industry or Tech Area: Wind Energy; Big Data Analytics & Simulations
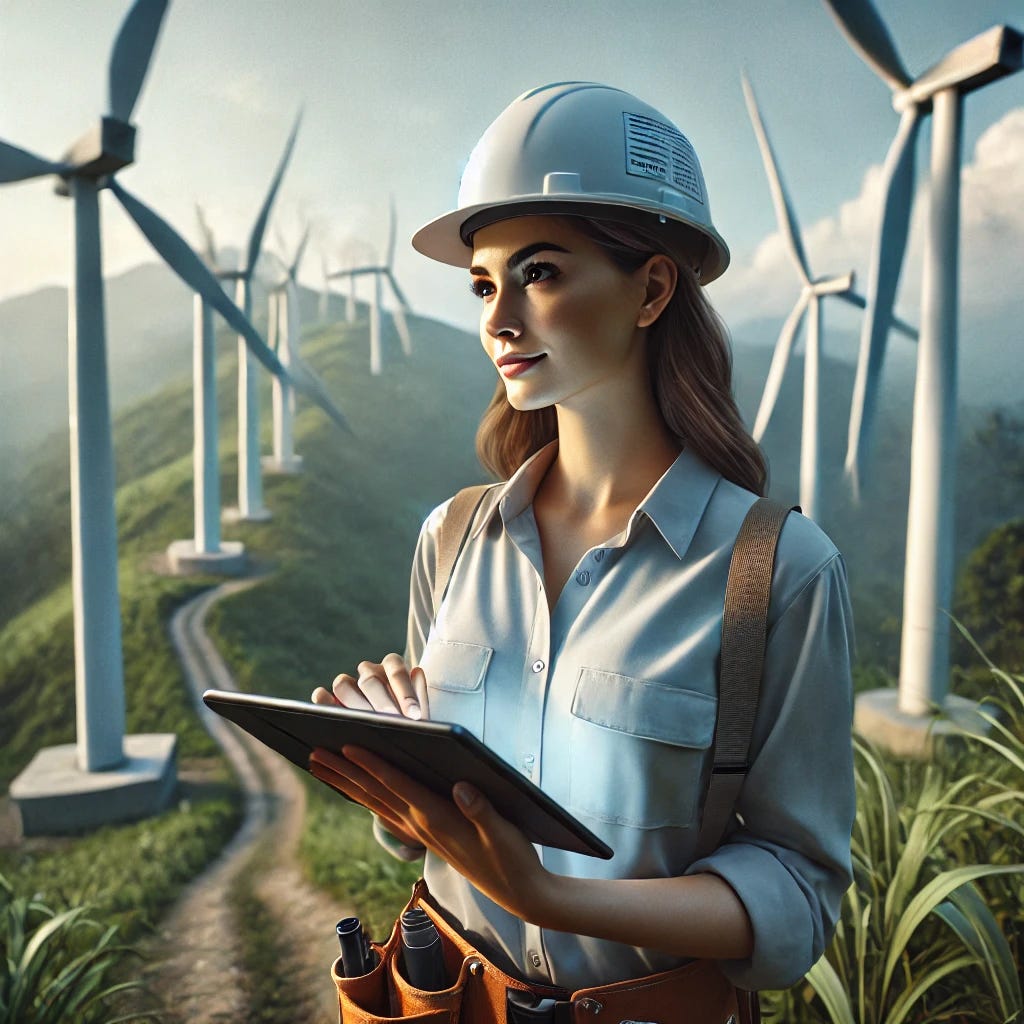