Memory Augmented Networks for Non-Stop Pedestrian Prediction
Continual Learning-Based Trajectory Prediction with Memory Augmented Networks
Tags: Universiti Malaya, Malaysia, Transportation & Automotive, Electronics & Robotics
This technology introduces CLTP-MAN, a continual learning-based method for trajectory prediction using memory-augmented neural networks. It addresses catastrophic forgetting, a challenge in deep neural networks when data distributions change over time. The system uses a memory module and sparse experience replay to retain past knowledge, improving generalization. It is particularly useful in applications like self-driving vehicles and social robots for accurate pedestrian trajectory forecasting. CLTP-MAN has shown improved performance in real-world data sets through external memory and multi-hop attention mechanisms.
IP Type or Form Factor: Discovery & Research; Software & Algorithm; Platform
TRL: 5 - prototype ready for testing in intended environment
Industry or Tech Area: Automobiles Autonomous; Robotics
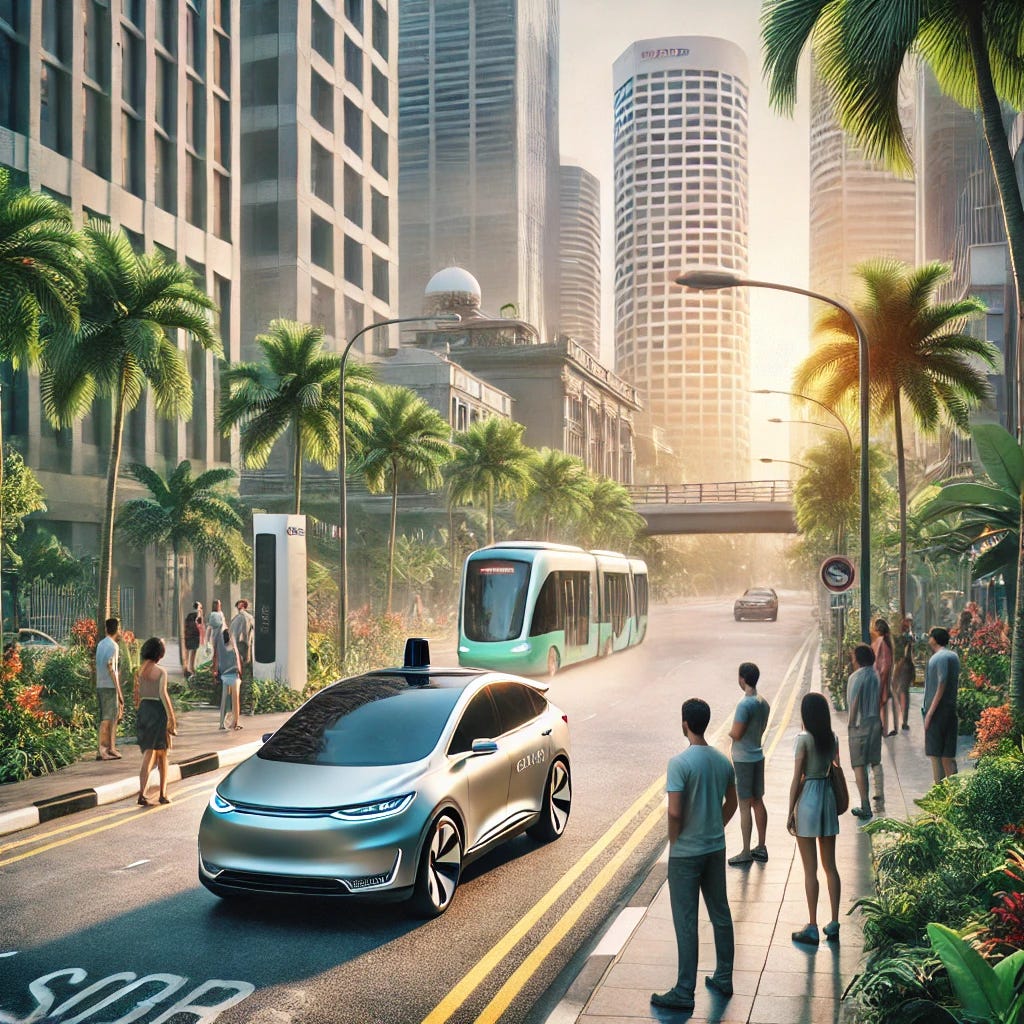